Thousands of employees analyzing your data
Artificial Intelligence (AI) is a human technology creation that is capable of making assumptions and take action by itself, making all these activities easier for you.
Example tasks in which this is done include speech recognition, computer vision, translation between (natural) languages, as well as other mappings of inputs.
The various sub-fields of AI research are centered around particular goals and the use of particular tools. The traditional goals of AI research include reasoning, knowledge representation, planning, learning, natural language processing, perception, and the ability to move and manipulate objects.
General intelligence (the ability to solve an arbitrary problem) is among the field’s long-term goals.
To solve these problems, AI researchers have adapted and integrated a wide range of problem-solving techniques – including search and mathematical optimization, formal logic, artificial neural networks, and methods based on statistics, probability and economics. AI also draws upon computer science, psychology, linguistics, philosophy, and many other fields.
And that means that all the data that tech companies are collecting are send to this AI data labeling companies that making profit by labeling your images, transcribing your audios and pinning your location, online shopping and browser history.
AI as technology is under development, but is already implemented in a lot of human activities.
Yes, your data is shared with thousands of employees of companies like Appen.
Most profit-maximizing algorithms, which underpin e-commerce sites, voice assistants, and self-driving cars, are based on deep learning, an AI technique that relies on scores of labeled examples to expand its capabilities.
The insatiable demand has created a need for a broad base of cheap labor to manually tag videos, sort photos, and transcribe audio.
The market value of sourcing and coordinating that “ghost work,” as it was memorably dubbed by anthropologist Mary Gray and computational social scientist Siddharth Suri, is projected to reach $13.7 billion by 2030.
For example, German car manufacturers, like Volkswagen and BMW, were panicked that the Teslas and Ubers of the world threatened to bring down their businesses. So they did what legacy companies do when they encounter fresh-faced competition: they wrote blank checks to keep up.
Like all AI models built on deep learning, self-driving cars need millions, if not billions, of labeled examples to be taught to “see.”
These examples come in the form of hours of video footage: every frame is carefully annotated to identify road markings, vehicles, pedestrians, trees, and trash cans for the car to follow or avoid. But unlike AI models that might categorize clothes or recommend news articles, self-driving cars require the highest levels of annotation accuracy. One too many mislabeled frames can be the difference between life and death.
For example on Appen.
They have multiple tech clients, like Roomba the cleaning robot.
Roomba saves images from each client and their home.
Appen has the task to analyzed and categorized the information from the images of millions of homes with roombas.
1. One browser shows running queue of tasks.
Each displays a title and an anonymized client ID, as well as the number of units it’s divided into and how much they can earn—usually cents—per unit.
The tasks range widely, from image tagging to content moderation to product categorization (say, determining whether an object in a photo falls under the heading “jewelry,” “clothing,” or “bags”).
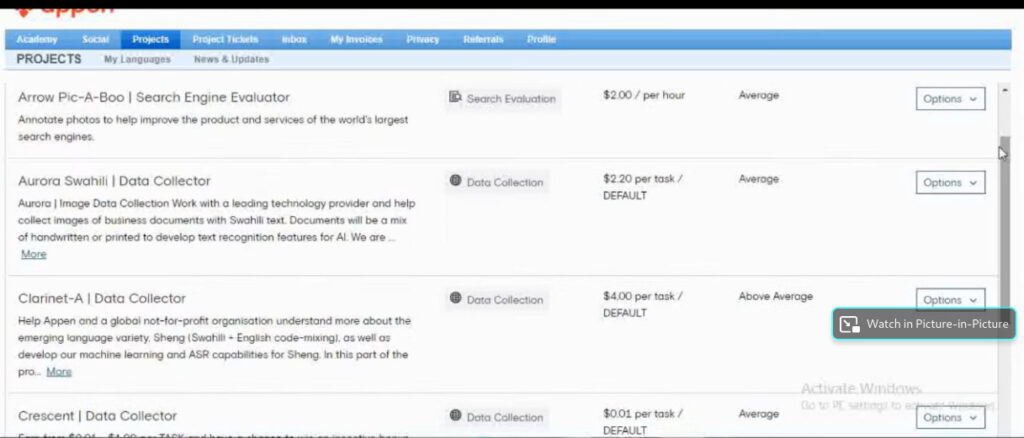
2. To claim a task, they click in, and the system presents the client’s instructions. Sometimes they’re clear; sometimes they’re not. Sometimes there are none at all.
One of the workers says that one of her tasks has proved impossible:
Fills her screen with a satellite image of a heavily forested area.
There are no instructions – just a button that says “tree” and “no trees” and a cursor that suggests she outline the appropriate parts of the image.
No matter which method she tries, her answer has been rejected every time. She is convinced that the client wants each tree – probably thousands – to be outlined individually.
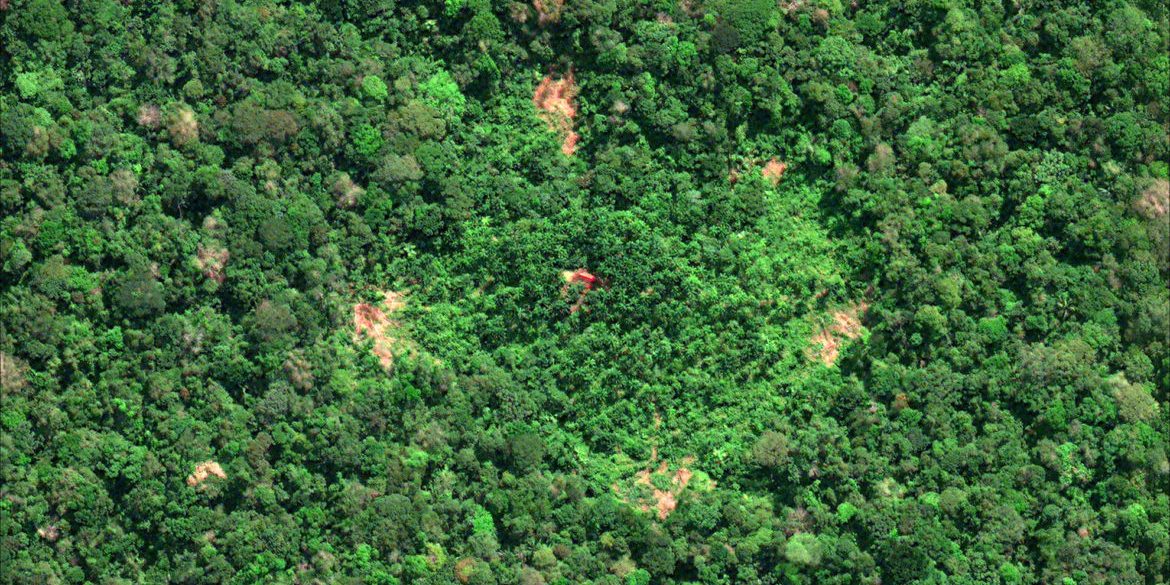
3. When they have completed the task, the earnings are displayed in pennies in the top right corner.
These industry were first full of cheap labour from Philippines, Kenya and Venezuela.
These were natural fits, with histories of outsourcing, populations that speak excellent English and, crucially, low wages.
By 2018, an estimated 200,000 Venezuelans had registered for Hive Micro and Spare5 another AI data labeling companies, making up 75% of their respective workforces.
Their tasks are simple, but require patience and time, and they have fun doing them. Some of these workers share pictures of what they do on social media. One of the most famous references was a photo of a woman on the toilet shared by a worker on the internet.
The pictures were taken by iRobot and are available in the Google search.
Artificial Intelligence (AI) is a powerful technology that has the potential to transform the way we live and work. AI systems can perform a range of tasks, from speech recognition and computer vision to natural language processing and perception.
However, to build these systems, AI researchers need vast amounts of labeled data, which is often provided by companies that specialize in data labeling services. These companies rely on a global workforce of cheap labor, mainly in developing countries, to manually tag videos, sort photos, and transcribe audio.
While this work can be tedious and low-paid, it is an essential part of the AI industry’s supply chain. The rise of AI data labeling services has created new challenges around privacy, security, and labor rights that need to be addressed. As AI continues to advance, we must ensure that it is developed and deployed ethically and responsibly to benefit all of humanity.
In Conclusion
AI can pose several digital privacy risks. One significant risk is that AI algorithms can process large amounts of personal data to draw inferences and predictions about individuals, even without their explicit consent or knowledge. For example, an AI system might use data from someone’s online searches, social media activity, or purchase history to make assumptions about their preferences, behavior, or even their health status.
Another risk is that AI systems can be vulnerable to security breaches, which can result in the theft or exposure of sensitive personal information. As AI becomes more prevalent in various sectors, including healthcare, finance, and government, the potential for data breaches and misuse of personal information increases.
Additionally, AI systems can perpetuate and amplify existing biases and discrimination, especially if they are trained on biased datasets or designed with biased algorithms. This can result in unfair treatment or exclusion of certain groups of people, which can have severe social and economic consequences.
Finally, the use of AI in surveillance and tracking can also raise concerns about privacy violations, particularly in authoritarian regimes where governments may use AI to monitor and suppress dissent.